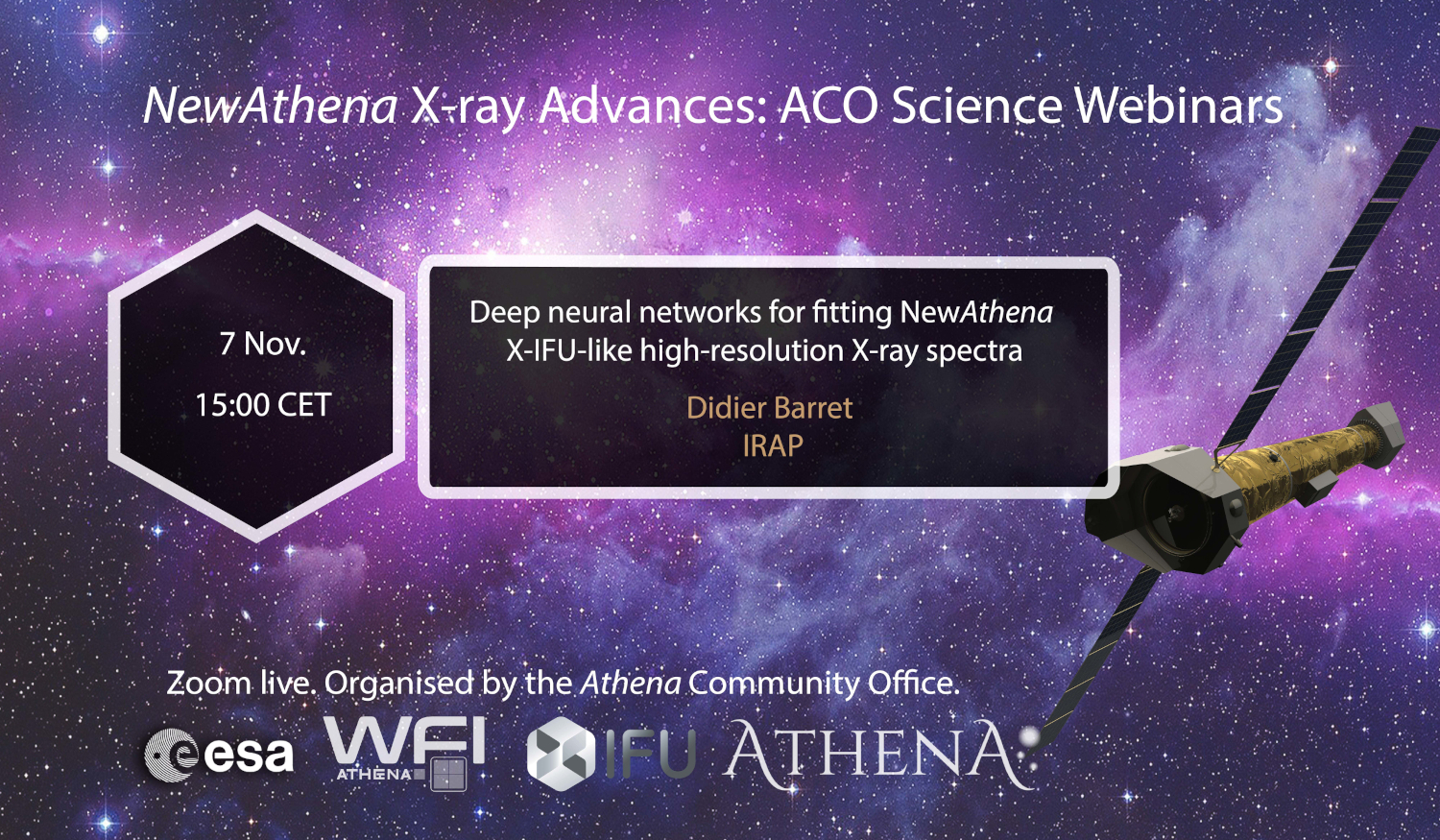
NewAthena X-ray Advances series: webinar by Didier Barret
On 7 November (15-16 CET), Didier Barret (Institut de Recherche en Astrophysique et Planétologie) will give the webinar entitled: Deep neural networks for fitting NewAthena X-IFU-like high-resolution X-ray spectra.
Abstract: In a recent paper [1], we applied a simulation-based inference with neural posterior estimation (SBI-NPE) to X-ray spectra. We trained a deep neural network with simulated spectra generated from a multiparameter source emission model folded through an instrument response, so that the network learns the mapping between the simulated spectra and their parameters and returns the posterior distribution. SBI-NPE was demonstrated to work equally well as standard X-ray spectral fitting, both in the Gaussian and Poisson regimes, on simulated and real data, yielding fully consistent results in terms of best-fit parameters and posterior distributions. The inference time is comparable to or smaller than the one needed for Bayesian inference when involving the computation of large Markov chain Monte Carlo chains to derive the posterior distributions. On the other hand, once properly trained, an amortized SBI-NPE network generates the posterior distributions in no time (less than 1 second per spectrum on a 6-core laptop). We showed that SBI-NPE is less sensitive to local minima trapping than standard fit statistic minimization techniques. With a simple model, we find that the neural network can be trained equally well on dimension-reduced spectra via a principal component decomposition, leading to a faster inference time with no significant degradation of the posteriors. Overall, we showed that simulation-based inference with neural posterior estimation can be considered as a complementary tool for X-ray spectral analysis, as the technique is robust and produces well-calibrated posterior distributions. In this talk, I will introduce the technique, show its applicability to fitting X-IFU like high-resolution X-ray spectra, introducing alternative upstream compression techniques (summary statistics, embedding networks), and finally considerations for including background. The Simulation based Inference X-ray Spectral Analysis (SIXSA) python package is available through GitHub, with the simulations performed with jaxspec [2]. The next release will be fully interfaced with PyXspec for generating the necessary simulations, thus giving access to the full suite of models implemented in XSPEC, and will enable SIXSA to be run via a command line.
The webinar connection details are as follows:
https://us06web.zoom.us/j/86291450611?pwd=K31DQXEXLK56vs3d08sUkKRlXs9aPZ.1 Passcode: 557900
Further information, including the code of conduct, is accessible on the dedicated website.
References:
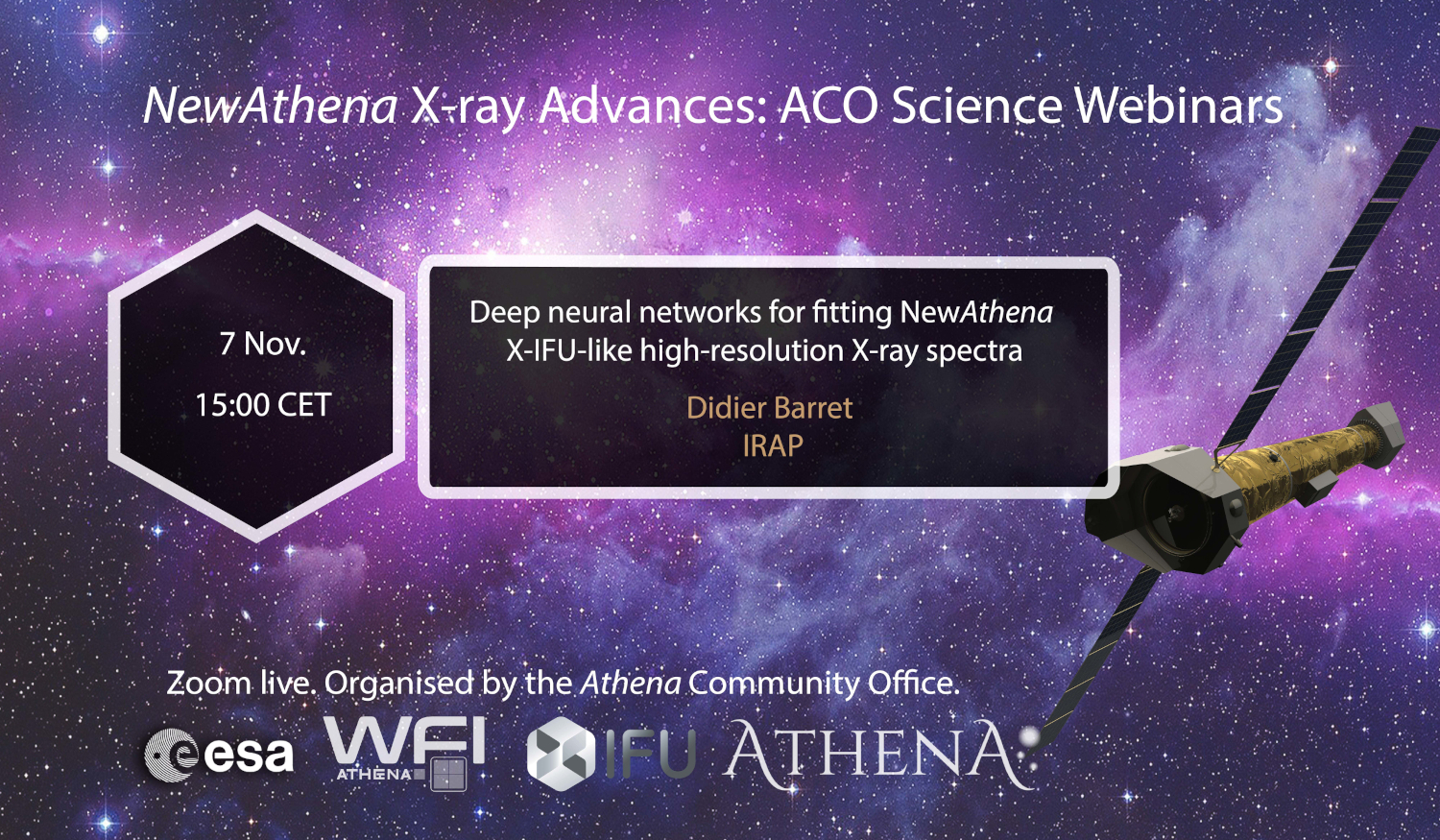
Latest News
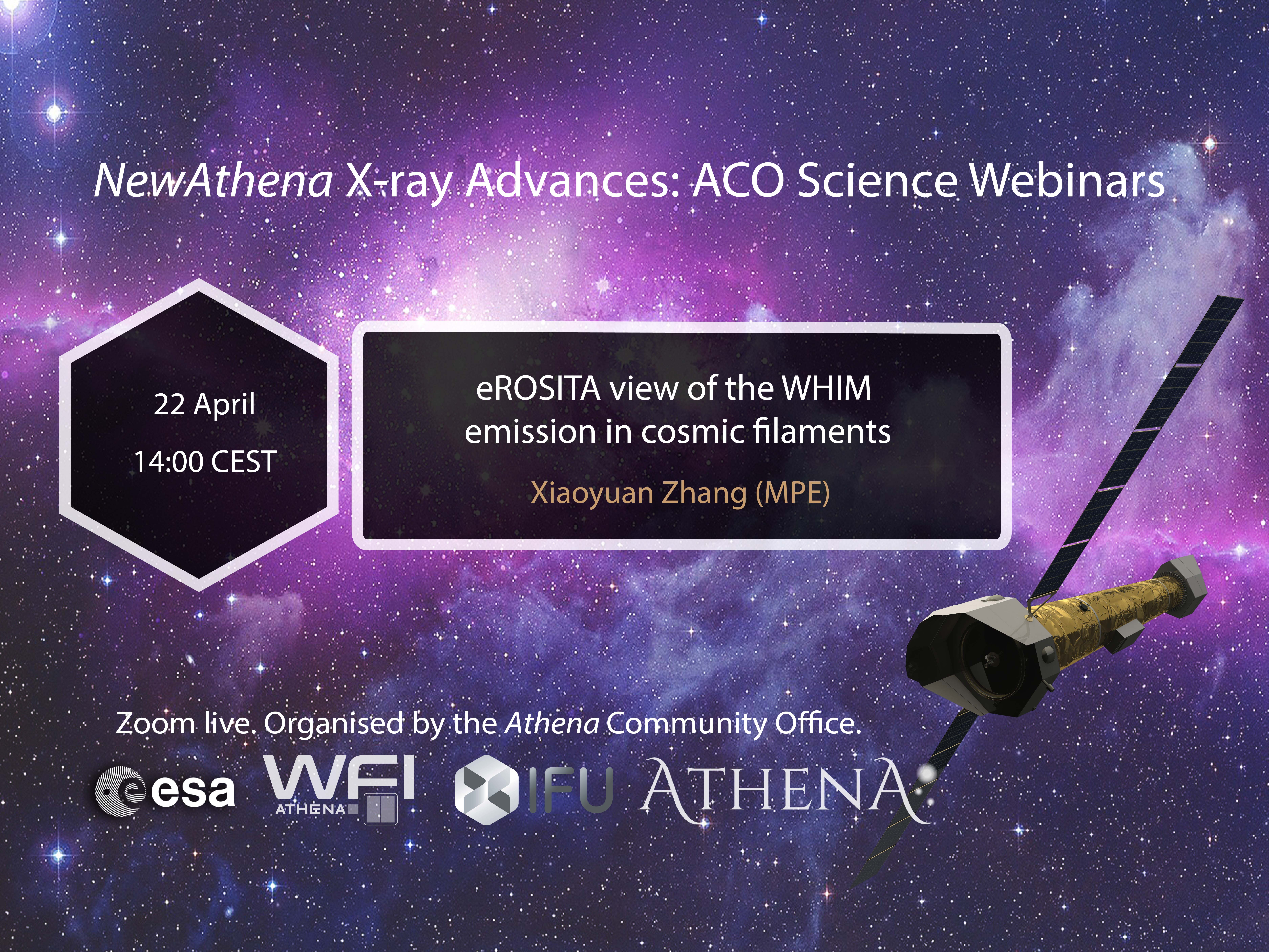
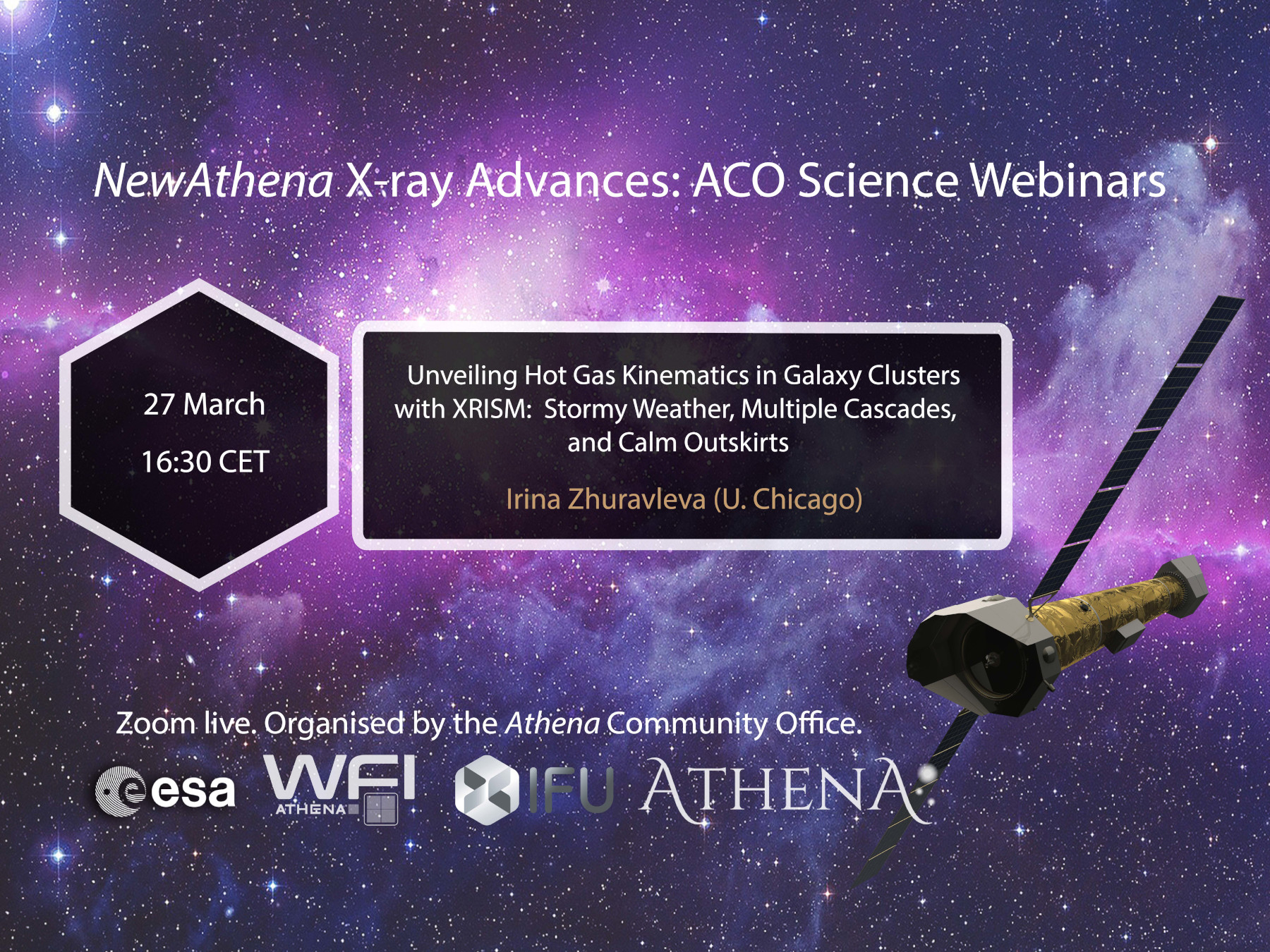
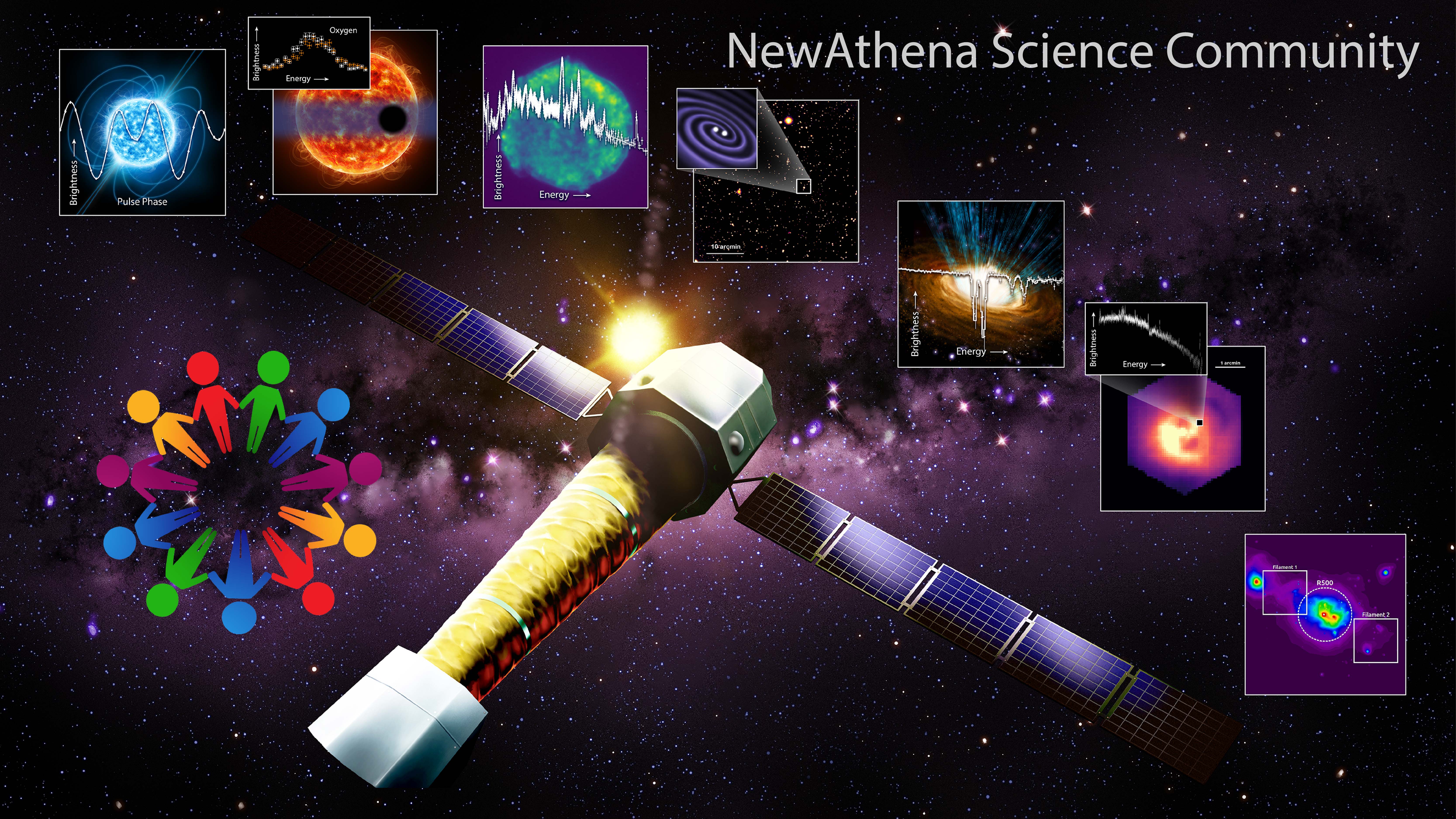
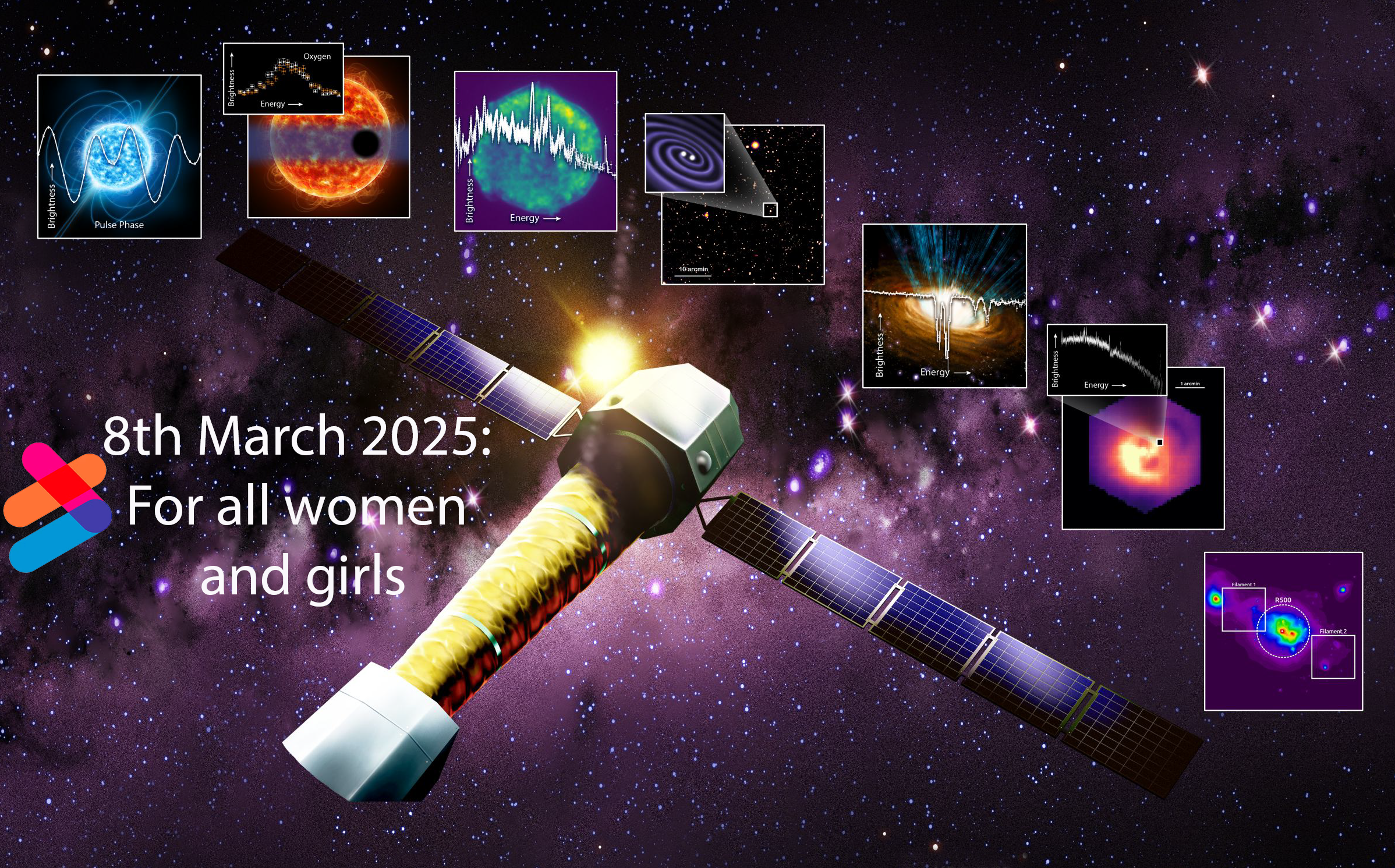
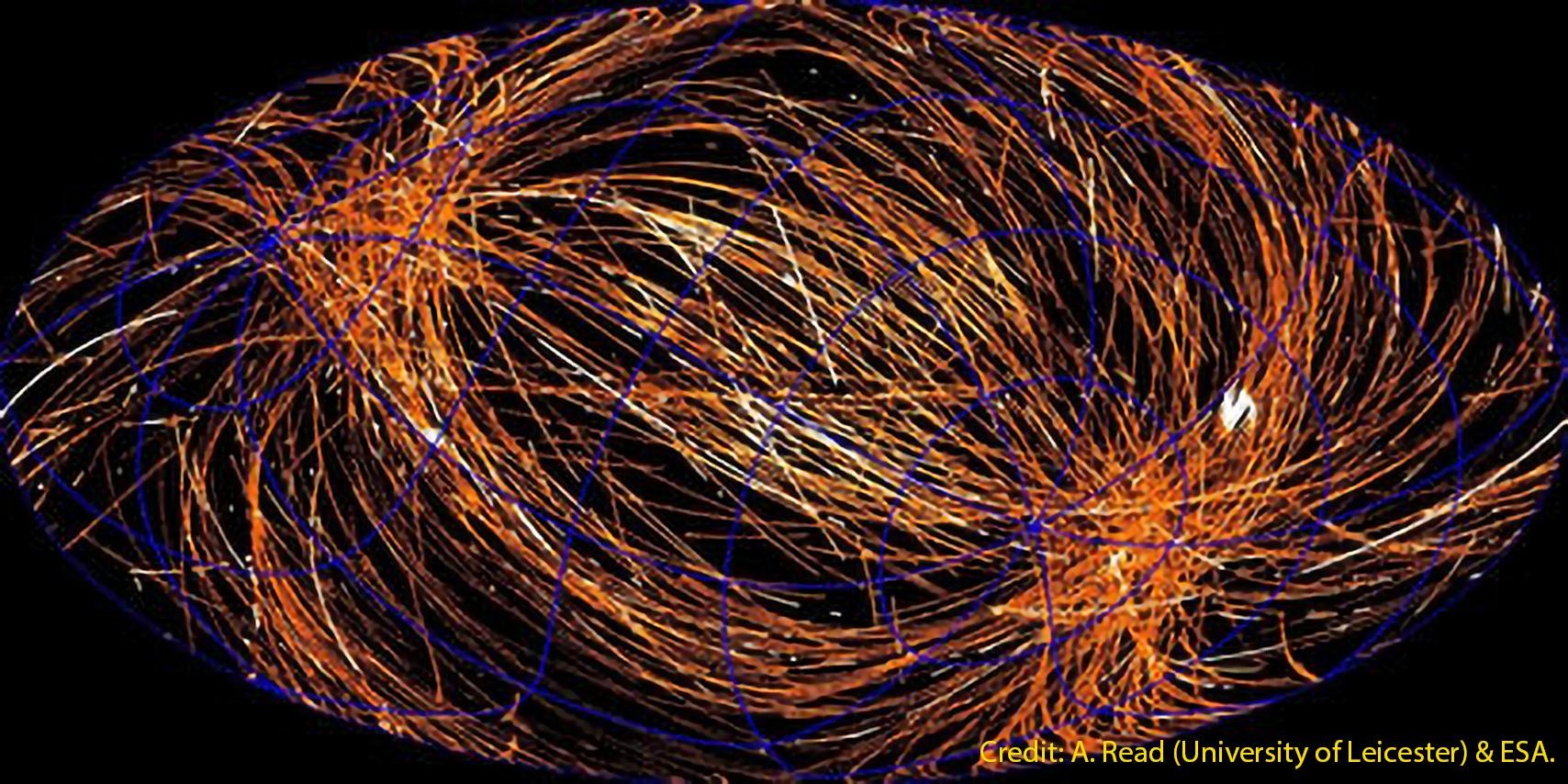
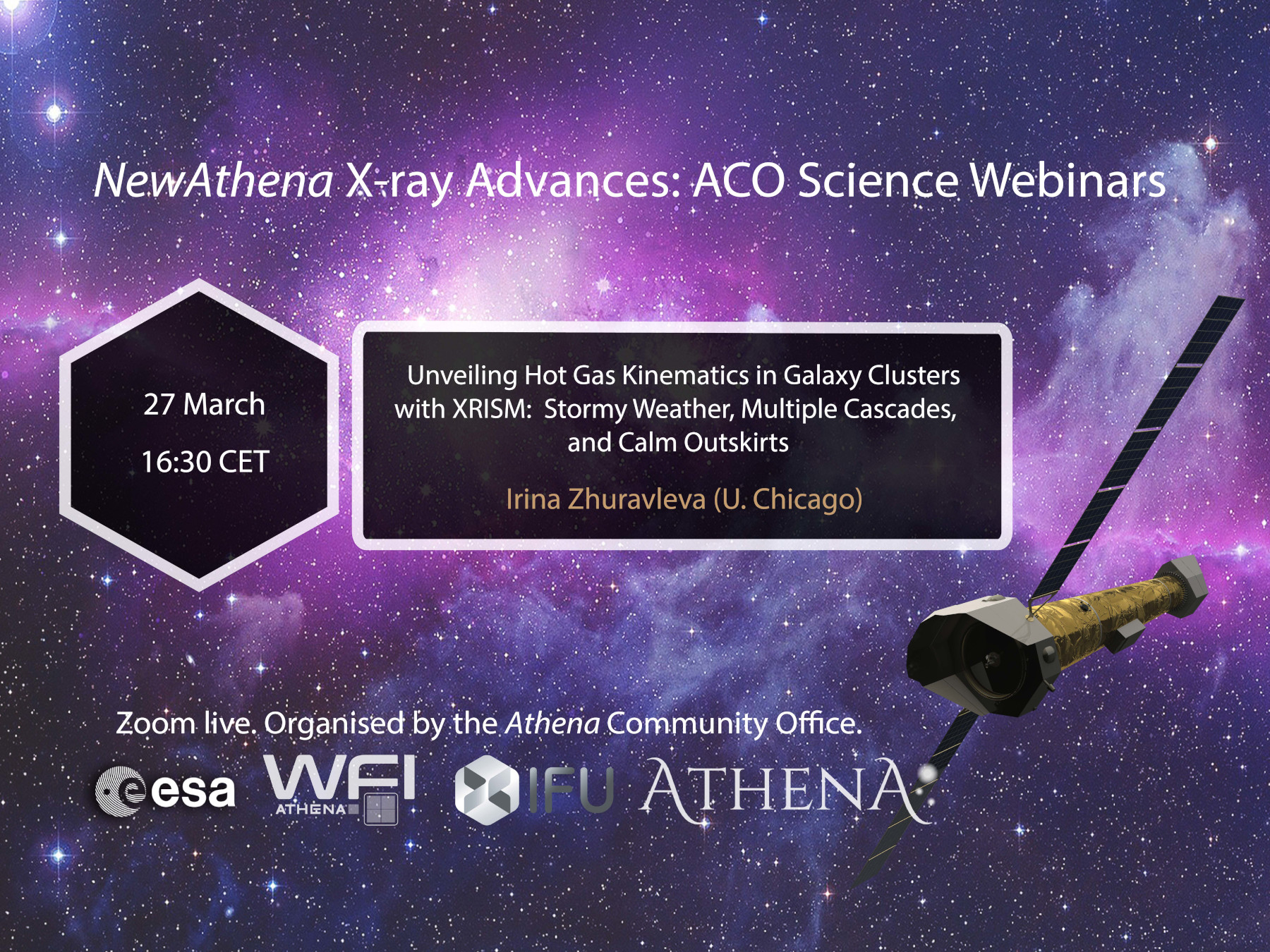
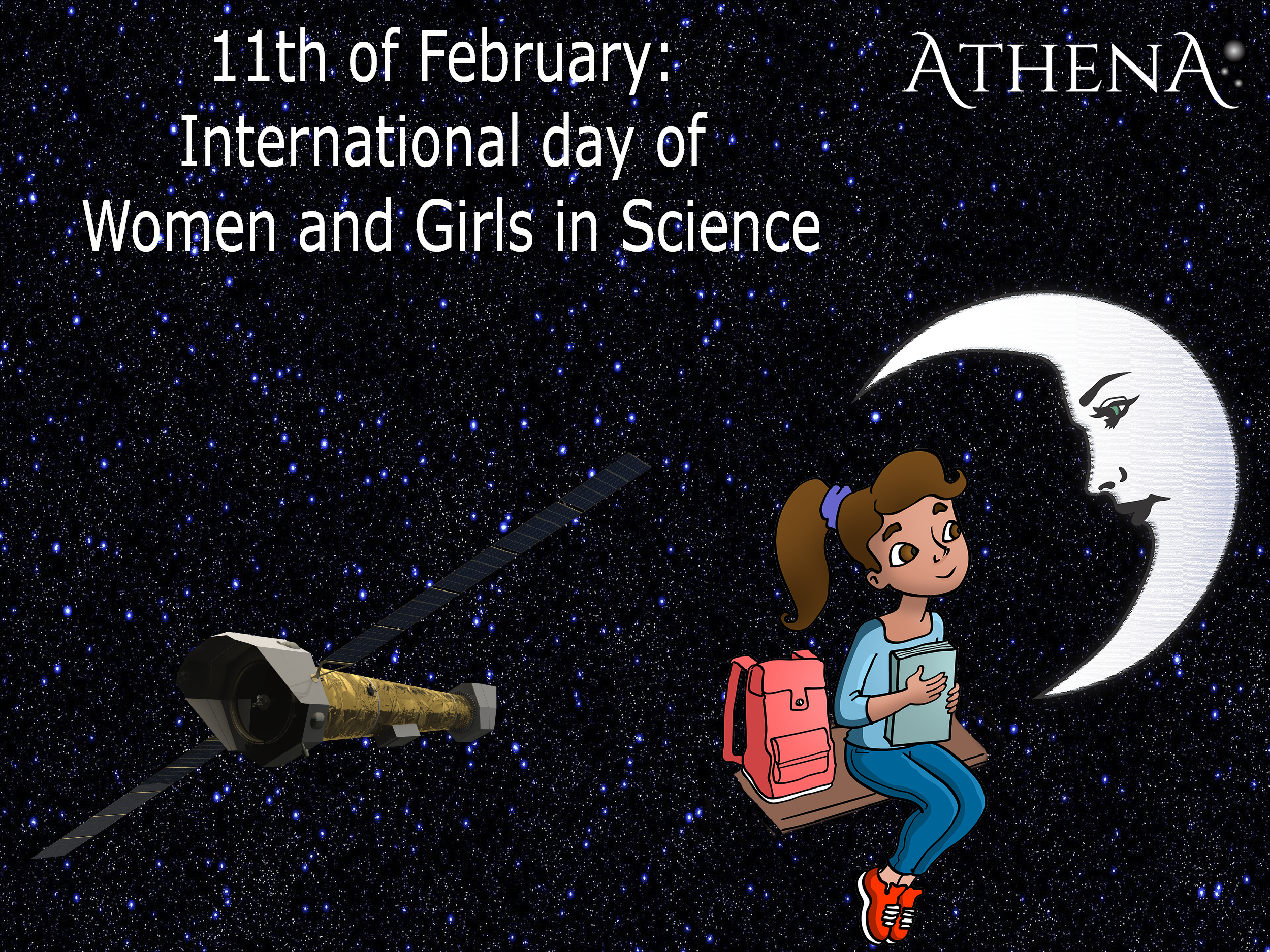
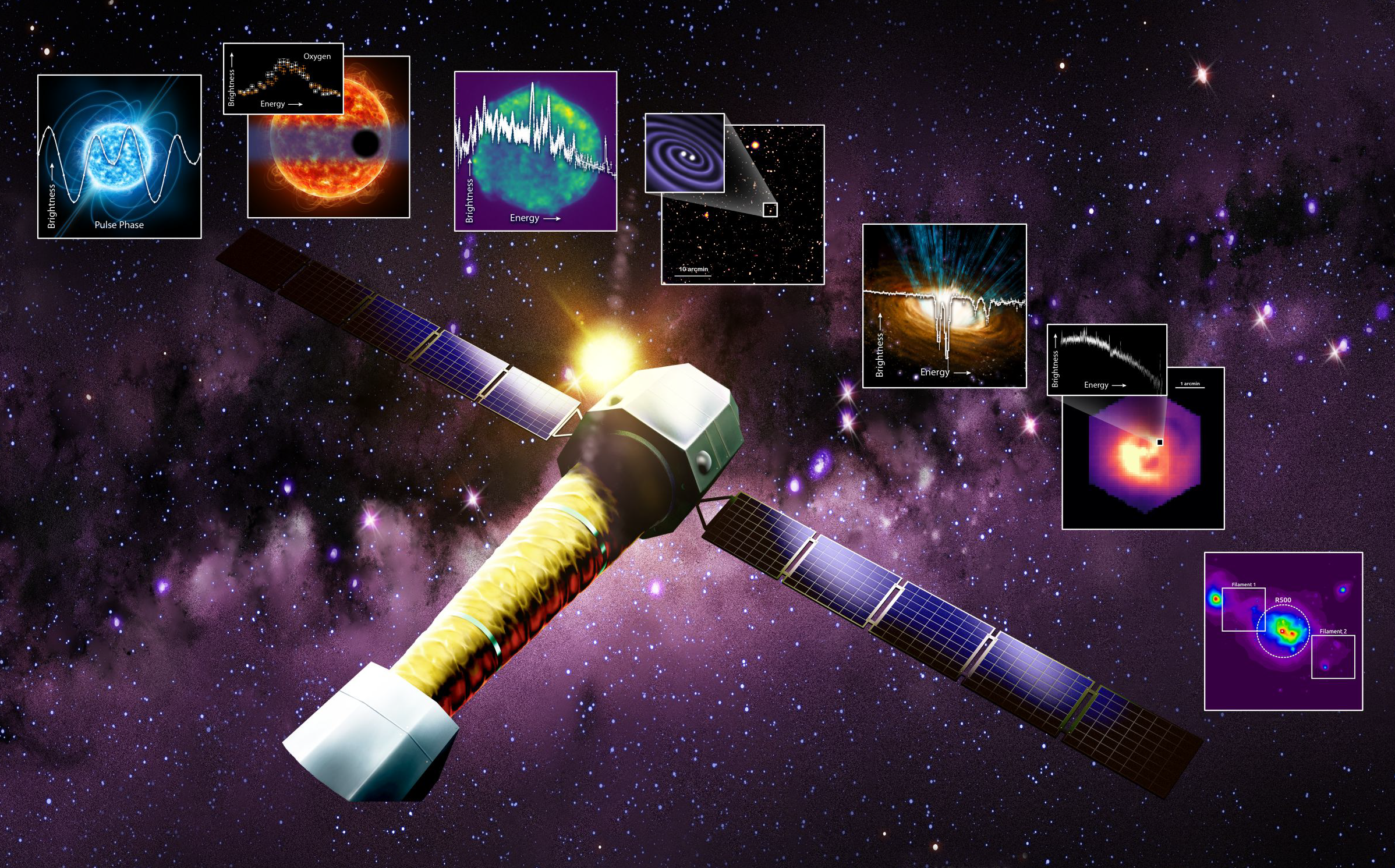